Hypertension, or high blood pressure, is a global health challenge affecting over 1.28 billion adults worldwide, according to the World Health Organization (WHO). Often dubbed the “silent killer,” hypertension typically presents no symptoms but significantly increases the risk of cardiovascular diseases, stroke, and kidney failure. Traditional methods of diagnosing and managing hypertension, while effective, are largely reactive, focusing on treating the condition after it develops. Recent advances in artificial intelligence (AI) are transforming this landscape by enabling predictive analytics to identify individuals at risk before hypertension manifests, paving the way for earlier intervention and improved health outcomes.
This article explores the role of AI in predicting hypertension risks, the mechanisms underlying predictive analytics, and the challenges and opportunities in leveraging these technologies for proactive healthcare.
You May Also Like: Can Meditation Really Lower Hypertension? What the Science Says
Understanding AI and Predictive Analytics
What Is Artificial Intelligence?
Artificial intelligence (AI) encompasses advanced algorithms and computational models that simulate human intelligence. AI applications range from analyzing large datasets to making predictions, automating processes, and supporting decision-making in healthcare and other fields.
Predictive Analytics in Healthcare
Predictive analytics uses statistical models and machine learning algorithms to analyze historical and real-time data, identifying patterns and correlations that can forecast future events. In hypertension management, predictive analytics leverages data from various sources—such as electronic health records (EHRs), wearable devices, and genetic profiles—to assess an individual’s likelihood of developing high blood pressure.
- Example: An AI-powered system might analyze trends in blood pressure readings, lifestyle factors, and biomarkers to predict whether a person is at risk for hypertension within the next five years.
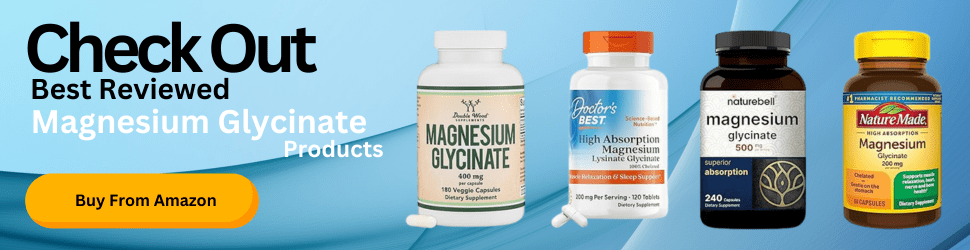
The Connection Between AI and Its Use in Predicting Hypertension
Why Predicting Hypertension Matters
Hypertension often develops over years, with subtle warning signs that may go unnoticed. Predictive tools powered by AI can detect these signs early, allowing for timely interventions to prevent or delay the onset of hypertension and reduce the associated risks of heart disease and stroke.
How AI Helps in Predicting Hypertension Risks
AI models analyze vast datasets, incorporating traditional risk factors such as age, weight, and family history, alongside non-traditional factors like stress levels, sleep quality, and environmental influences. This comprehensive approach enables AI systems to provide nuanced risk assessments.
- Evidence: A study published in The Lancet Digital Health (2021) demonstrated that AI algorithms achieved 85% accuracy in predicting hypertension onset by analyzing patient records and lifestyle data.
Applications of AI in Predicting Hypertension Risks
1. Early Identification of At-Risk Individuals
AI systems excel at processing complex data sets from multiple sources, identifying patterns that human clinicians might overlook. These insights enable healthcare providers to pinpoint individuals at risk before symptoms develop.
- Example: AI analyzes subtle fluctuations in blood pressure trends recorded over time, flagging patients who may be in the prehypertensive stage.
2. Real-Time Monitoring and Feedback
Wearable devices like smartwatches and fitness trackers collect continuous data on metrics such as heart rate, physical activity, and sleep. AI-powered analytics process this data in real time to provide actionable feedback.
- Case Study: A fitness tracker equipped with AI detects irregularities in a user’s resting heart rate, suggesting elevated stress levels that could contribute to hypertension. The device recommends relaxation techniques or prompts the user to consult a healthcare professional.
3. Personalized Prevention Strategies
AI generates individualized risk profiles based on a combination of genetic, lifestyle, and environmental factors. These profiles guide personalized interventions, such as tailored dietary recommendations, exercise plans, or stress management techniques.
- Example: A patient with a genetic predisposition to salt sensitivity receives specific guidance on reducing sodium intake to prevent hypertension.
4. Population Health Management
AI tools allow healthcare systems to stratify populations by risk level, enabling targeted outreach and preventive measures for high-risk groups. This proactive approach reduces the burden on healthcare systems and improves outcomes at a community level.
- Evidence: A predictive analytics initiative implemented in a hospital network reduced hypertension-related hospitalizations by 20% within three years, according to research published in JAMA Internal Medicine (2019).
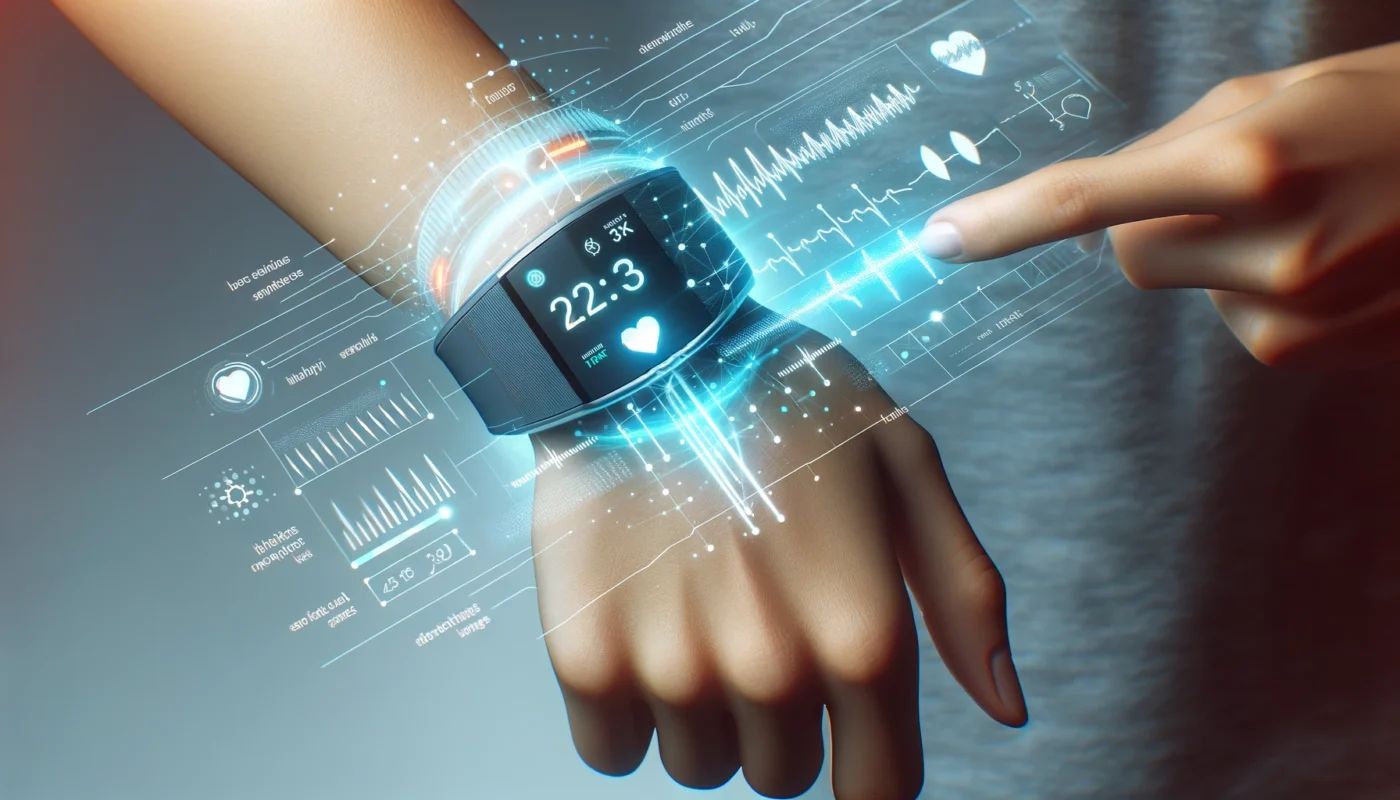
Innovations in AI for Hypertension Management
1. Deep Learning Algorithms
Deep learning models are highly effective at identifying complex patterns in large datasets. In hypertension prediction, these models analyze variables such as blood pressure trends, biomarkers, and lifestyle data to generate precise risk assessments.
- Case Study: Researchers at Stanford University developed a deep learning algorithm that predicted hypertension onset with 90% accuracy using multi-modal data from EHRs and wearable devices.
2. Natural Language Processing (NLP)
NLP tools extract valuable insights from unstructured data, such as clinical notes, patient-reported symptoms, and lifestyle descriptions. This additional layer of information enhances the accuracy of hypertension risk predictions.
- Example: An NLP-based system flags patients with documented complaints of chronic stress or poor sleep, identifying individuals at higher risk for hypertension.
3. Wearable Technology and Sensors
Innovative wearable devices, such as smartwatches with cuffless blood pressure monitoring, enable continuous data collection and real-time feedback.
- Innovation: AI-powered wearables analyze blood pressure trends and other physiological markers, alerting users to seek medical advice if significant deviations are detected.
Challenges in Implementing AI for Predicting Hypertension
1. Data Quality and Integration
AI models rely on high-quality data from diverse sources to make accurate predictions. However, incomplete or inconsistent data can compromise the reliability of these systems.
- Solution: Standardizing data collection methods and integrating data from EHRs, wearables, and laboratory tests can enhance AI performance.
2. Ethical and Privacy Concerns
The use of personal health data in AI systems raises ethical issues related to privacy, security, and algorithmic bias. Ensuring patient consent and data anonymization is critical.
- Solution: Robust data governance frameworks and transparency in AI algorithms can address these concerns.
3. Access and Equity
AI technologies may not be equally accessible to all populations, particularly in low-resource settings. This digital divide could exacerbate existing health disparities.
- Solution: Expanding access to affordable AI tools and providing education on their use can help bridge this gap.
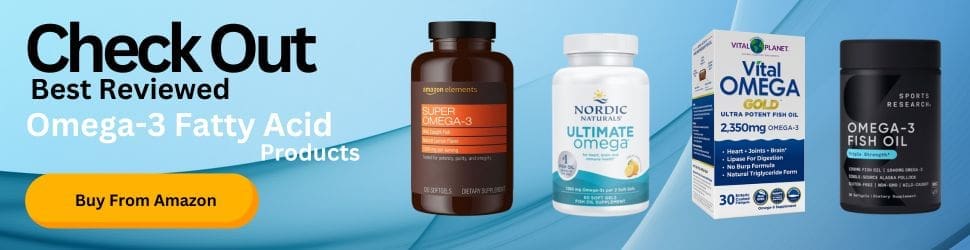
The Role of Supplements in Supporting Hypertension Prevention
In addition to AI-powered interventions, nutritional supplements can play a complementary role in hypertension prevention. Below are five evidence-based supplements for blood pressure management:
1. Omega-3 Fatty Acids
Omega-3s reduce inflammation and improve endothelial function, supporting cardiovascular health. A meta-analysis in Hypertension (2018) reported reductions in SBP by 4 mmHg with omega-3 supplementation.
2. Coenzyme Q10 (CoQ10)
CoQ10 is an antioxidant that protects blood vessels from oxidative damage and supports energy production in cells. A trial in Hypertension Research (2007) demonstrated that CoQ10 supplementation lowered SBP by 11 mmHg.
3. Magnesium Glycinate
Magnesium helps relax blood vessels, improving blood flow and reducing vascular resistance. A study in Magnesium Research (2016) found that magnesium supplementation reduced systolic blood pressure (SBP) by 5 mmHg.
4. Hibiscus Extract
Hibiscus contains bioactive compounds that enhance nitric oxide availability, improving vascular relaxation. Research in The Journal of Nutrition (2010) showed that hibiscus tea reduced SBP by 6 mmHg.
5. Beetroot Powder
Beetroot is rich in nitrates, which are converted to nitric oxide to improve blood vessel function and lower blood pressure. A study in Nutrition Journal (2017) found that beetroot supplementation reduced SBP by 4 mmHg.
The Future of AI in Hypertension Prevention
Precision Medicine and Biomarker Integration
Advances in biomarker research combined with AI algorithms could offer even more precise risk assessments, enabling hyper-targeted interventions for hypertension prevention.
Real-Time Monitoring and Feedback Loops
The integration of AI with wearable devices and mobile health apps offers the potential for continuous, real-time monitoring of hypertension risks, transforming prevention into a dynamic, personalized process.
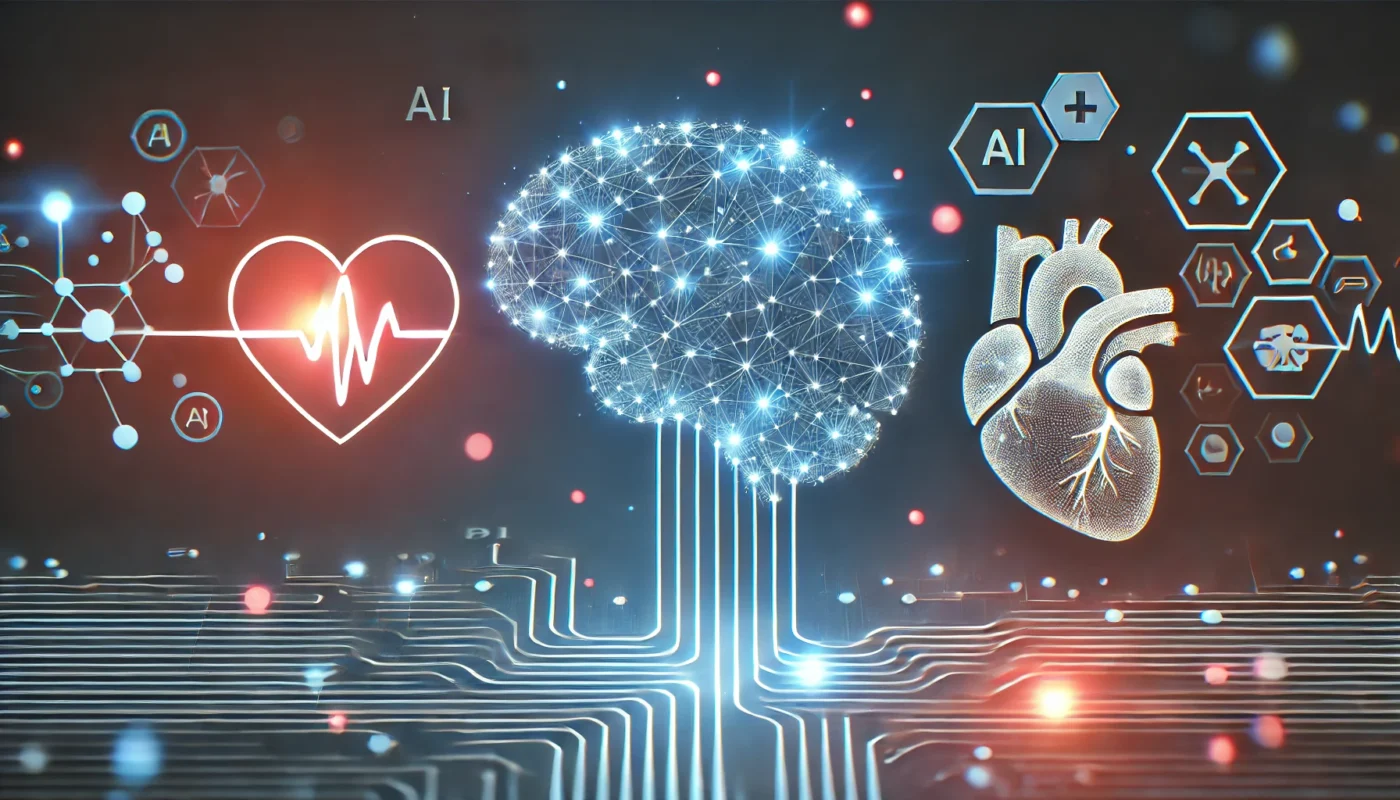
Conclusion
AI-powered predictive analytics has the potential to revolutionize hypertension management by identifying individuals at risk and enabling early intervention. By analyzing complex datasets and incorporating non-traditional risk factors, AI provides nuanced insights that traditional methods cannot. While challenges such as data quality and ethical concerns must be addressed, the integration of AI with wearable technology, lifestyle interventions, and nutritional supplements offers a comprehensive approach to hypertension prevention. As research and innovation continue, AI is poised to play a central role in reducing the global burden of hypertension and its associated complications.
References
- The Lancet Digital Health. (2021). AI models in predicting hypertension: A review. The Lancet Digital Health. Retrieved from https://www.thelancet.com
- JAMA Internal Medicine. (2019). Population health management with predictive analytics. JAMA Internal Medicine. Retrieved from https://jamanetwork.com
- Hypertension Research. (2007). Coenzyme Q10 and its effects on blood pressure. Hypertension Research. Retrieved from https://www.nature.com
- The Journal of Nutrition. (2010). Effects of hibiscus tea on vascular function. The Journal of Nutrition. Retrieved from https://academic.oup.com
- Nutrition Journal. (2017). Beetroot supplementation and blood pressure reduction. Nutrition Journal. Retrieved from https://www.biomedcentral.com
Key TERMS for this article:
Artificial Intelligence, Hypertension Prediction, Predictive Analytics, Blood Pressure Management, Wearable Technology, Cardiovascular Health, Nutritional Supplements
Relevant and useful TAGS for this article:
Hypertension, Artificial Intelligence, Predictive Analytics, Blood Pressure Monitoring, Wearable Devices, Cardiovascular Wellness, Health Technology, Early Intervention, Nutritional Support, Precision Medicine
Important Note: The information contained in this article is for general informational purposes only, and should not be construed as health or medical advice, nor is it intended to diagnose, prevent, treat, or cure any disease or health condition. Before embarking on any diet, fitness regimen, or program of nutritional supplementation, it is advisable to consult your healthcare professional in order to determine its safety and probable efficacy in terms of your individual state of health.
Regarding Nutritional Supplements Or Other Non-Prescription Health Products: If any nutritional supplements or other non-prescription health products are mentioned in the foregoing article, any claims or statements made about them have not been evaluated by the U.S. Food and Drug Administration, and such nutritional supplements or other health products are not intended to diagnose, treat, cure, or prevent any disease.