Hypertension, or high blood pressure, is a global health crisis affecting more than 1.28 billion adults worldwide, according to the World Health Organization (WHO). Despite decades of medical advancements, the condition remains a leading risk factor for cardiovascular diseases, stroke, and kidney failure. However, the advent of big data analytics is revolutionizing how hypertension is studied, diagnosed, and managed. By analyzing vast amounts of healthcare data, researchers and clinicians are uncovering patterns, predicting risks, and tailoring treatments with unprecedented precision. This article explores how data-driven approaches are transforming hypertension care, improving patient outcomes, and paving the way for a more personalized and proactive healthcare system.
You May Also Like: Can Artificial Organs Help with Hypertension? The Case for Bioengineering
Understanding Big Data in Healthcare
What Is Big Data?
Big data in healthcare refers to the massive volumes of structured and unstructured data generated from various sources, including:
- Electronic Health Records (EHRs): Patient demographics, clinical notes, and treatment histories.
- Wearable Devices: Continuous monitoring of blood pressure, heart rate, and physical activity.
- Genomic Data: Information about genetic predispositions to hypertension.
- Claims and Billing Data: Insights into healthcare utilization and costs.
- Population Health Surveys: Epidemiological data about hypertension prevalence and risk factors.
Characteristics of Big Data
Big data is often described using the “5 Vs”:
- Volume: The sheer size of the datasets.
- Velocity: The speed at which data is generated and processed.
- Variety: The diversity of data types and sources.
- Veracity: The reliability and accuracy of the data.
- Value: The actionable insights derived from the data.
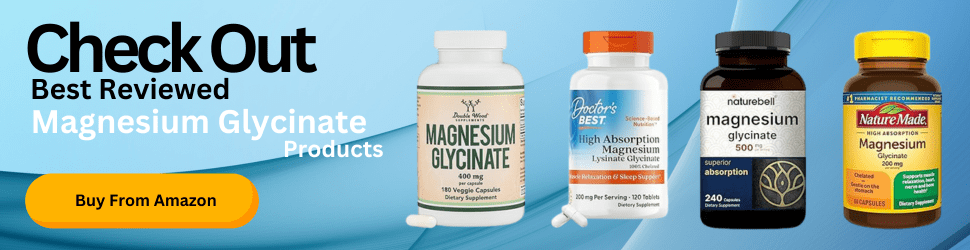
Big Data and Hypertension: Applications and Benefits
Big data analytics is transforming how hypertension is understood and managed. Below are some of its key applications:
1. Predictive Modeling for Hypertension Risk
By analyzing patient data from EHRs, wearable devices, and genetic profiles, predictive algorithms can identify individuals at high risk for developing hypertension. These models consider a range of factors, including lifestyle habits, family history, and social determinants of health.
- Example: A study in Hypertension Research (2021) demonstrated that machine learning algorithms using data from 10,000 patients accurately predicted hypertension onset with 85% sensitivity.
2. Personalized Treatment Plans
Data analytics enables clinicians to tailor hypertension treatments based on an individual’s unique characteristics. By integrating data from genomics, pharmacology, and patient preferences, big data supports precision medicine approaches.
- Example: Genomic data can help identify patients who are more likely to respond to specific antihypertensive medications, such as ACE inhibitors or calcium channel blockers.
3. Real-Time Monitoring and Early Intervention
Wearable devices, such as smartwatches and blood pressure monitors, continuously collect data on blood pressure and related metrics. Big data platforms analyze this information in real time, alerting patients and healthcare providers to abnormal trends.
- Evidence: A study in Journal of Medical Internet Research (2020) found that real-time monitoring through wearable devices reduced hypertension-related hospitalizations by 15%.
4. Population Health Management
By aggregating data from millions of patients, public health organizations can identify patterns and trends in hypertension prevalence and treatment effectiveness. This information guides policy decisions and resource allocation.
- Example: Data from population-level studies helped the U.S. Centers for Disease Control and Prevention (CDC) implement targeted hypertension screening campaigns in high-risk communities.
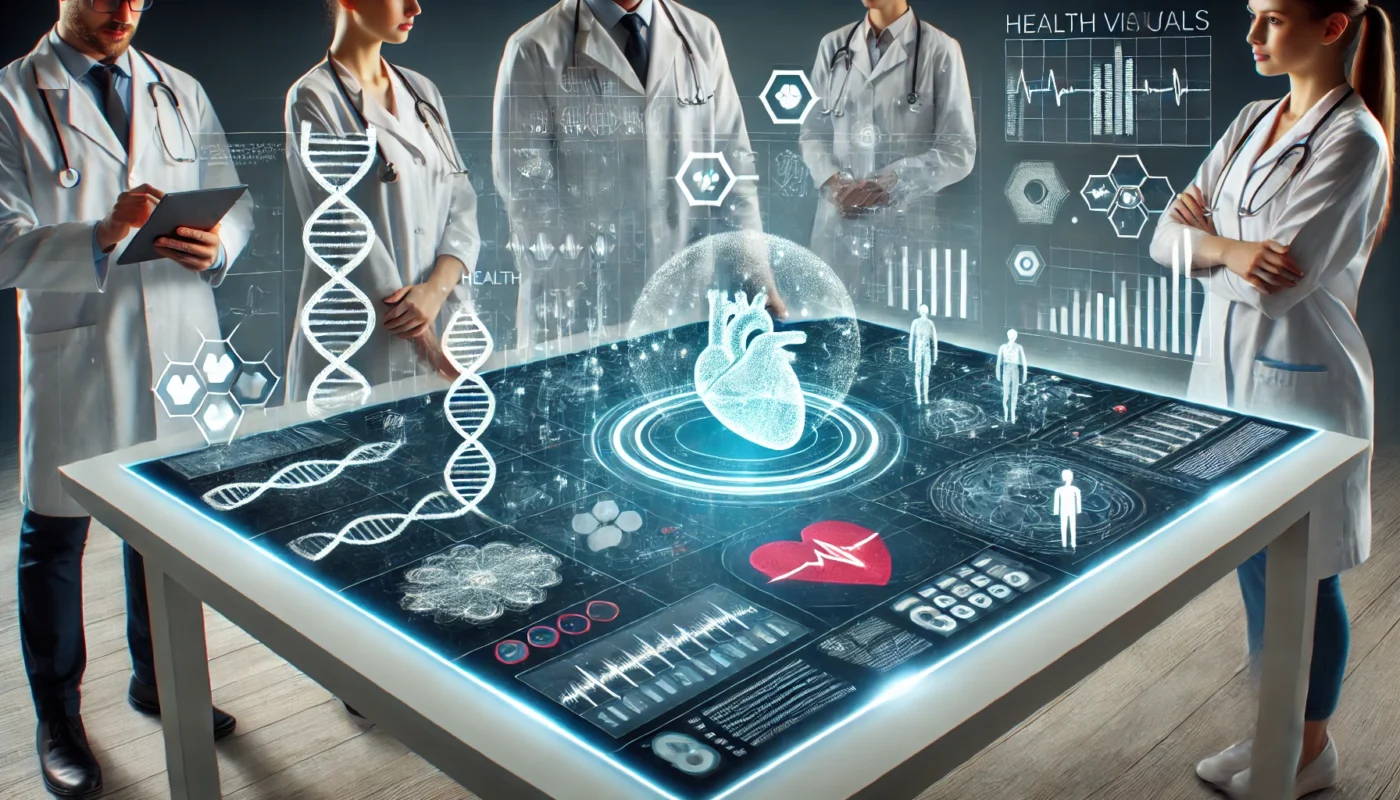
Challenges in Using Big Data for Hypertension
While the potential of big data is immense, several challenges must be addressed to fully realize its benefits:
1. Data Privacy and Security
Handling sensitive patient information requires robust data protection measures. Breaches can compromise patient trust and violate regulations like the Health Insurance Portability and Accountability Act (HIPAA).
2. Data Integration
Healthcare data is often siloed across different systems, making it challenging to integrate and analyze comprehensively. Interoperability standards are essential to unify data sources.
3. Algorithm Bias
Predictive models can reflect biases present in the training data, leading to unequal treatment recommendations. For instance, underrepresented populations may not receive accurate risk assessments.
4. Interpretation Complexity
The vast amount of data generated by big data systems requires specialized expertise for interpretation. Clinicians and researchers need advanced training in data science and machine learning.
Case Studies: Big Data in Hypertension Management
1. Hypertension Insights from Genomic Data
A large-scale genomic study published in Nature Genetics (2019) analyzed the DNA of 500,000 individuals to identify genetic markers associated with hypertension. The study uncovered over 200 genetic loci linked to blood pressure regulation, offering new targets for drug development.
2. AI-Driven Clinical Decision Support
A healthcare system in Singapore implemented an AI-powered decision support tool that analyzed patient data to recommend antihypertensive medications. The system improved medication adherence by 20% and reduced adverse drug reactions.
3. Wearable Technology in Remote Patient Monitoring
In a pilot program in the United Kingdom, patients with uncontrolled hypertension were provided with wearable blood pressure monitors. Data from these devices was transmitted to a centralized platform, where alerts were sent to clinicians for timely interventions. The program reduced hypertension complications by 18% over 12 months.
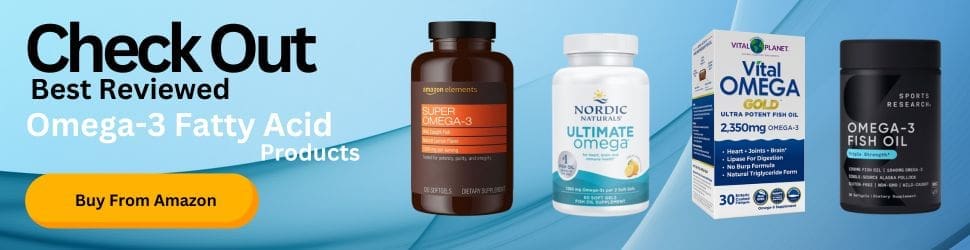
Role of Nutritional Supplements in Hypertension Management
Big data has also advanced our understanding of the role of nutritional supplements in hypertension. Below are five evidence-based supplements supported by data analytics:
1. Omega-3 Fatty Acids
Omega-3s improve endothelial function and reduce inflammation, key factors in hypertension management. A meta-analysis in Hypertension (2018) found a reduction of 4 mmHg in SBP with omega-3 supplementation.
2. Magnesium Glycinate
Magnesium supports vascular relaxation and improves blood pressure control. A meta-analysis in Magnesium Research (2016) reported a 5 mmHg reduction in systolic blood pressure (SBP) with magnesium supplementation.
3. Hibiscus Extract
Hibiscus contains bioactive compounds that promote nitric oxide production, enhancing vascular health. A study in The Journal of Nutrition (2010) demonstrated that hibiscus tea reduced SBP by 6 mmHg.
4. Coenzyme Q10 (CoQ10)
CoQ10 reduces oxidative stress and improves mitochondrial function, supporting cardiovascular health. A clinical trial in Hypertension Research (2007) found that CoQ10 supplementation lowered SBP by 11 mmHg.
5. Beetroot Powder
Beetroot is rich in nitrates, which improve blood flow and lower blood pressure. A study in Nutrition Journal (2017) showed a reduction in SBP by 4 mmHg with beetroot supplementation.
The Future of Big Data in Hypertension
1. Integration with Artificial Intelligence
AI will enhance big data analytics by identifying complex patterns and making real-time predictions. AI-driven systems could recommend personalized interventions based on continuous data input from wearable devices and EHRs.
2. Precision Public Health
Big data will enable targeted public health interventions, such as community-specific education campaigns and resource allocation to high-risk populations.
3. Digital Twins
Digital twins—virtual models of patients—will use big data to simulate treatment outcomes. This approach will help clinicians test interventions in a risk-free environment before applying them to patients.
4. Collaborative Platforms
Healthcare organizations, governments, and tech companies are increasingly collaborating to create unified data ecosystems. Initiatives like the All of Us Research Program in the U.S. aim to gather data from diverse populations to improve hypertension care globally.
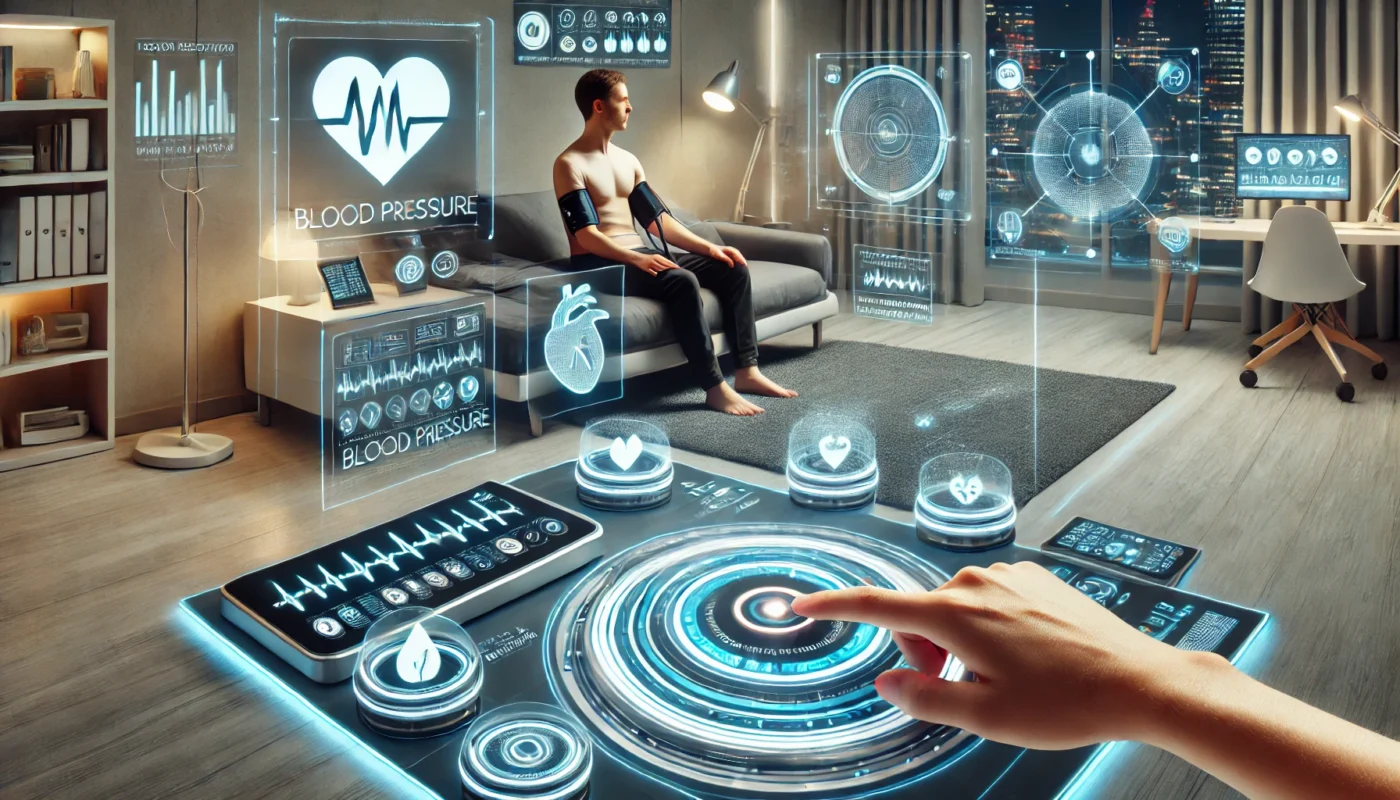
Conclusion
Big data analytics is revolutionizing how hypertension is understood and managed. By leveraging data from EHRs, wearable devices, and population studies, researchers and clinicians can identify patterns, predict risks, and develop personalized treatment plans. Despite challenges such as data privacy and integration, the potential of big data to improve patient outcomes is immense. As technology advances, big data will play an increasingly central role in hypertension care, enabling more precise, proactive, and equitable healthcare solutions. The future of hypertension management lies in harnessing the power of data to deliver better outcomes for millions of patients worldwide.
References
- Hypertension Research. (2021). Machine learning in hypertension risk prediction: A study on 10,000 patients. Hypertension Research. Retrieved from https://www.nature.com/hr
- Nature Genetics. (2019). Genetic markers for blood pressure regulation: Insights from a large-scale study. Nature Genetics. Retrieved from https://www.nature.com/ng
- Journal of Medical Internet Research. (2020). Wearable devices and hypertension management: Real-time monitoring outcomes. Journal of Medical Internet Research. Retrieved from https://www.jmir.org
- Magnesium Research. (2016). The effects of magnesium supplementation on blood pressure: A meta-analysis. Magnesium Research. Retrieved from https://www.springer.com
- Nutrition Journal. (2017). Beetroot supplementation and blood pressure control: A clinical study. Nutrition Journal. Retrieved from https://www.biomedcentral.com
Key TERMS for this article:
Hypertension, Big Data, Predictive Analytics, Wearable Technology, Precision Medicine, Population Health, AI in Healthcare
Relevant and useful TAGS for this article:
Hypertension, Big Data, Machine Learning, Wearable Devices, Precision Medicine, AI Healthcare, Cardiovascular Health, Population Health Analytics, Digital Health, Predictive Modeling
Important Note: The information contained in this article is for general informational purposes only, and should not be construed as health or medical advice, nor is it intended to diagnose, prevent, treat, or cure any disease or health condition. Before embarking on any diet, fitness regimen, or program of nutritional supplementation, it is advisable to consult your healthcare professional in order to determine its safety and probable efficacy in terms of your individual state of health.
Regarding Nutritional Supplements Or Other Non-Prescription Health Products: If any nutritional supplements or other non-prescription health products are mentioned in the foregoing article, any claims or statements made about them have not been evaluated by the U.S. Food and Drug Administration, and such nutritional supplements or other health products are not intended to diagnose, treat, cure, or prevent any disease.